James Garcia
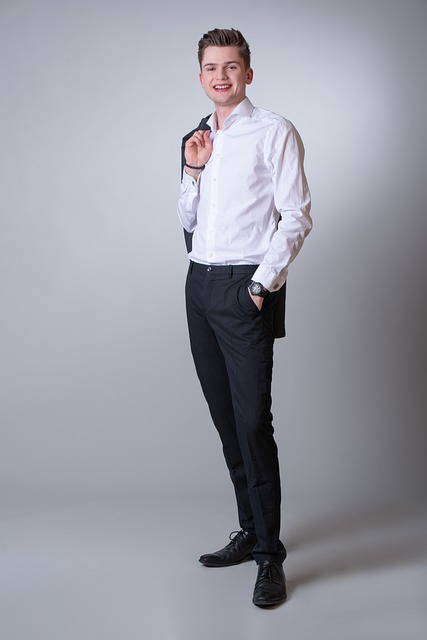
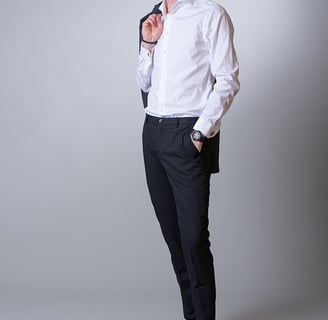
Name: James
Role: Dynamic Pricing Specialist
Expertise: AI-Driven Real-Time Price Optimization Based on Market Demand
Professional Summary:
James is a dynamic professional in the field of AI-driven dynamic pricing, specializing in developing systems that real-time adjust product prices based on market demand, competitor behavior, and consumer trends. With a strong foundation in data science, machine learning, and economics, James is dedicated to helping businesses maximize revenue, optimize inventory, and enhance customer satisfaction through intelligent pricing strategies. His work empowers companies to stay competitive in fast-changing markets by leveraging AI to make data-driven pricing decisions.
Key Competencies:
AI-Powered Dynamic Pricing Models:
Develops advanced machine learning algorithms to analyze market demand, competitor pricing, and customer behavior, enabling real-time price adjustments.
Utilizes predictive analytics to forecast demand fluctuations and optimize pricing strategies accordingly.
Revenue Optimization:
Designs pricing frameworks that balance profitability with customer acquisition and retention, ensuring long-term business growth.
Implements A/B testing and continuous learning mechanisms to refine pricing models and maximize revenue.
Market Demand Analysis:
Proficient in integrating and analyzing data from multiple sources, including sales history, competitor data, and economic indicators, to uncover actionable insights.
Builds scalable data pipelines to process and interpret large datasets for real-time pricing decisions.
Cross-Functional Collaboration:
Collaborates with marketing, sales, and finance teams to align pricing strategies with organizational goals.
Provides training and support to ensure the successful adoption of dynamic pricing tools.
Ethical Pricing Practices:
Ensures pricing strategies are transparent, fair, and compliant with regulatory standards, fostering trust with customers.
Advocates for ethical AI practices in dynamic pricing to avoid exploitative or discriminatory outcomes.
Career Highlights:
Developed an AI-driven dynamic pricing system that increased revenue by 15% for a leading e-commerce platform.
Designed a real-time pricing framework that reduced inventory overstock by 20% for a global retail chain.
Published influential research on AI applications in dynamic pricing, earning recognition at international tech and business conferences.
Personal Statement:
"I am passionate about leveraging AI to create intelligent pricing strategies that drive business success while delivering value to customers. My mission is to develop dynamic pricing systems that adapt to market changes in real-time, ensuring competitiveness and sustainability."
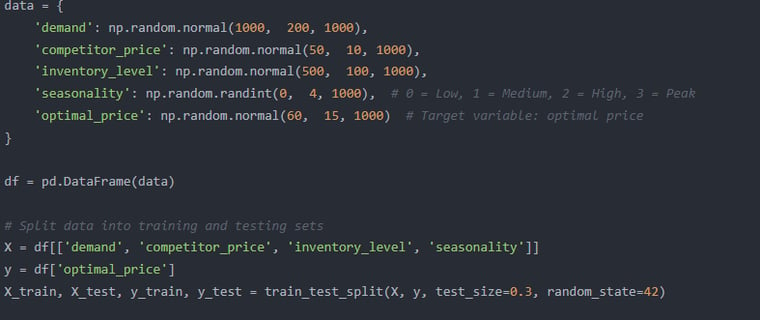
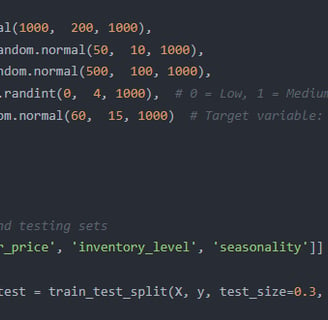
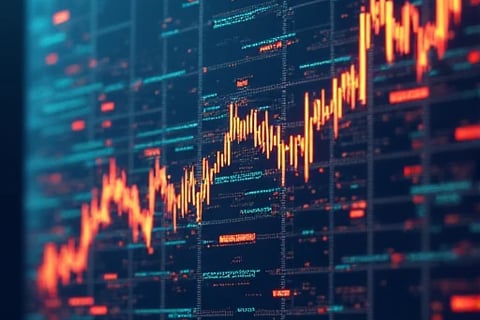
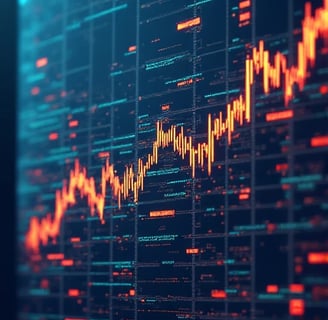
Expected Outcomes
This research aims to demonstrate that fine-tuning GPT-4 can significantly enhance its ability to optimize dynamic pricing strategies. The outcomes will contribute to a deeper understanding of how advanced AI models can be adapted for real-time pricing applications. Additionally, the study will highlight the societal impact of AI in improving business profitability, enhancing customer experience, and advancing the field of data-driven decision-making in pricing.
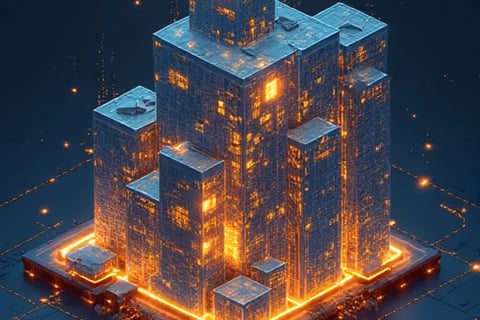
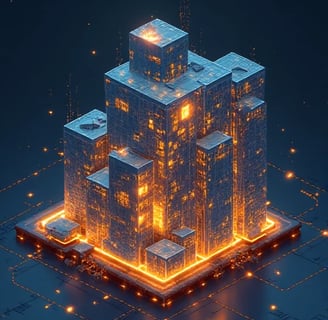
Fine-Tuning Necessity
Fine-tuning GPT-4 is essential for this research because publicly available GPT-3.5 lacks the specialized capabilities required for analyzing complex market data and generating optimal pricing strategies. Dynamic pricing involves highly domain-specific knowledge, intricate demand patterns, and nuanced pricing criteria that general-purpose models like GPT-3.5 cannot adequately address. Fine-tuning GPT-4 allows the model to learn from dynamic pricing datasets, adapt to the unique challenges of the domain, and provide more accurate and actionable insights. This level of customization is critical for advancing AI’s role in dynamic pricing and ensuring its practical utility in real-world business scenarios.
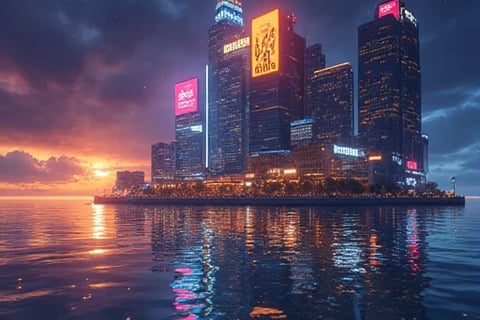
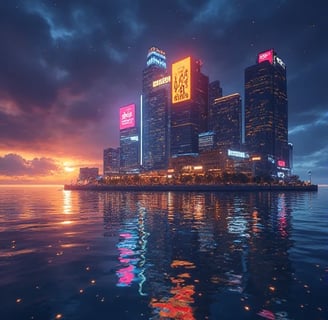
Past Research
To better understand the context of this submission, I recommend reviewing my previous work on the application of AI in dynamic pricing, particularly the study titled "Enhancing Pricing Strategies Using Machine Learning Models." This research explored the use of reinforcement learning and predictive analytics for improving pricing accuracy. Additionally, my paper "Adapting Large Language Models for Domain-Specific Applications in Dynamic Pricing" provides insights into the fine-tuning process and its potential to enhance model performance in specialized fields.